New Model Transforms Multiple Myeloma Risk Prediction
A team of researchers has developed a powerful new tool that could transform how doctors treat multiple myeloma, a complex and often unpredictable blood cancer.
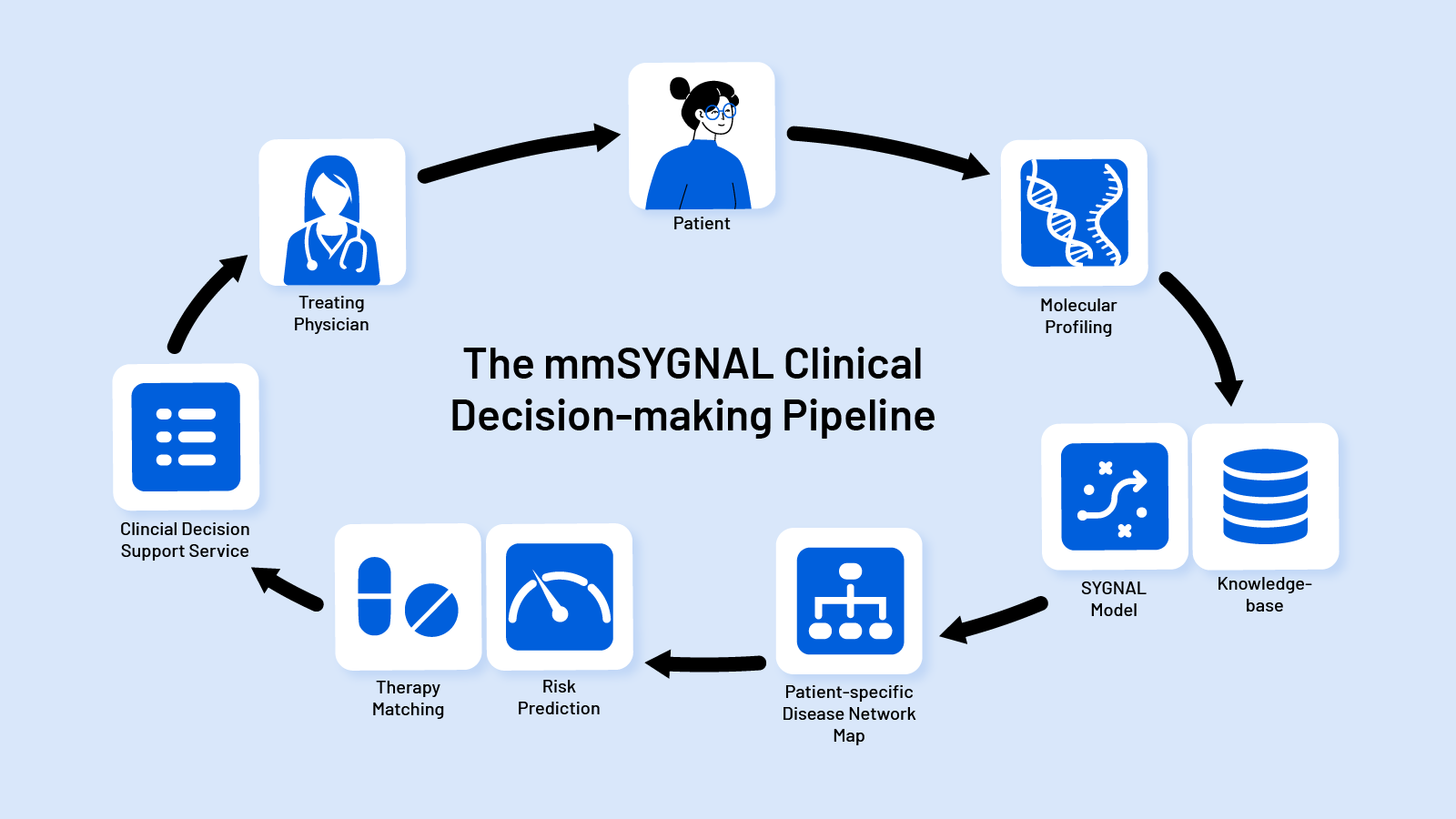
A team of researchers has developed a powerful new tool that could transform how doctors treat multiple myeloma, a complex and often unpredictable blood cancer. The model, called mmSYGNAL, uses machine learning and systems biology to analyze how genes interact in cancer cells, providing more accurate risk predictions and helping identify the most effective treatments for each patient. The research was published in the British Journal of Cancer.
While current diagnostic tools rely on detecting genetic mutations and chromosomal abnormalities, they often fail to explain why two patients with the same mutation can have vastly different outcomes. mmSYGNAL fills that gap by mapping the molecular network driving the disease, and using gene expression to offer insight into the risk of cancer progression and predicting which treatments are more likely to be effective.
“Two patients might have the same high-risk mutation, but very different outcomes,” said Dr. Carl Murie, lead researcher on the project and a research scientist in ISB’s Baliga Lab. “Our model explains why, and could help doctors make better treatment decisions based on that deeper understanding.”
Understanding mmSYGNAL
mmSYGNAL – short for SYstems Genetic Network AnaLysis – analyzes how genes behave and interact across thousands of cancer samples. The ISB team trained the model on data from 881 multiple myeloma patients, incorporating gene expression, genome sequencing, chromosomal changes, and transcription factor activity. Using the model and tumor profile (gene expression or RNA-seq) from a patient, the model pinpoints the biological programs actively driving disease progression in that patient. Remarkably, ISB scientists demonstrated that the model accurately predicts the risk of disease progression not only at the time of primary diagnosis but also after relapse, transplant, or multiple rounds of treatment. Importantly, in addition to predicting which patients are at high or extreme risk of progressing, the model also predicts which treatments are likely to be most effective in each patient.
Key Findings from the Study
1. More Accurate Risk Stratification
mmSYGNAL significantly outperformed standard tools like ISS, R-ISS, and commercial gene panels (SKY92, GEP70) in predicting the risk of disease progression. In one striking example, patients with the same high-risk mutation, known as t(4;14), were shown to have vastly different outcomes based on their underlying biology. Some had a median progression-free survival (PFS) of just five months, while others had up to 30 months.
Traditional models would lump these patients into the same risk group. mmSYGNAL reveals the deeper biological differences that truly matter for patient care.
2. Works Across All Stages of Disease
The team validated mmSYGNAL in five independent patient groups, including those that were newly diagnosed and those with relapsed disease. It consistently provided more accurate predictions of disease progression and treatment response, regardless of when the cancer was profiled.
3. Predicting Drug Sensitivity Up Front
Perhaps most exciting is mmSYGNAL’s ability to help predict which drugs might work best before treatment even begins. By analyzing which biological programs are active in a patient’s cancer cells, the model can infer whether that patient is likely to respond to specific therapies, including those still in clinical trials.
“This is a big step forward,” said ISB Professor and Director Dr. Nitin Baliga, senior author of the paper. “Traditional machine learning models struggle to make these predictions due to a lack of large training datasets. But our approach accurately predicted drug sensitivity in eight relapsed patients across a panel of 67 drugs.”
New Hope for High-Risk Patients
The researchers identified 25 key transcriptional programs that appear to drive disease progression. Many of these include known or investigational drug targets, giving high-risk patients access to treatment strategies that weren’t previously considered.
For example, patients flagged as “extreme-risk” by mmSYGNAL showed increased sensitivity to new classes of PARP inhibitors, proteasome inhibitors, and immunomodulatory agents, offering new therapeutic options where few existed before.
Real-World Applications
The implications of mmSYGNAL are wide-reaching:
- Smarter treatment decisions: Doctors can use the model to determine how aggressive initial treatment should be – and how to adjust it over time.
- Personalized care: By identifying drugs that are most likely to work based on tumor biology, clinicians can make more informed choices from a growing list of approved therapies.
- Better clinical trials: mmSYGNAL can help match patients with trials that are most likely to benefit them, improving trial outcomes and speeding up drug development.
The model can even help salvage failed trials by identifying why a small group of patients responded, pointing the way to future success.
Moving Toward Truly Personalized Medicine
By combining machine learning with deep biological insight, mmSYGNAL represents a major advance in the quest for personalized cancer treatment. Unlike conventional models that focus only on static mutations, mmSYGNAL captures the dynamic and evolving nature of cancer, revealing how dysfunction in specific genes and pathways propagates through gene networks to drive the progression of the disease.
“We believe this model has the potential to revolutionize multiple myeloma care,” Murie said. “It gives clinicians a much clearer picture of a patient’s disease and how to treat it starting from day one.”
The ISB team continues to refine and expand mmSYGNAL and is working toward broader clinical adoption.
“The more data we gather, the smarter and more useful this model becomes,” Baliga said. “It’s a glimpse into the future of personalized precision medicine.”