A New Technology for Personalized Cancer Care
Every cancer patient’s disease is different. Yet most cancer patients receive a similar set of aggressive treatments resulting in high treatment failure rate and many adverse side effects. By deeply characterizing a patient’s risk of progression, the Baliga Lab’s SYGNAL technology helps physicians select personalized treatment plans.
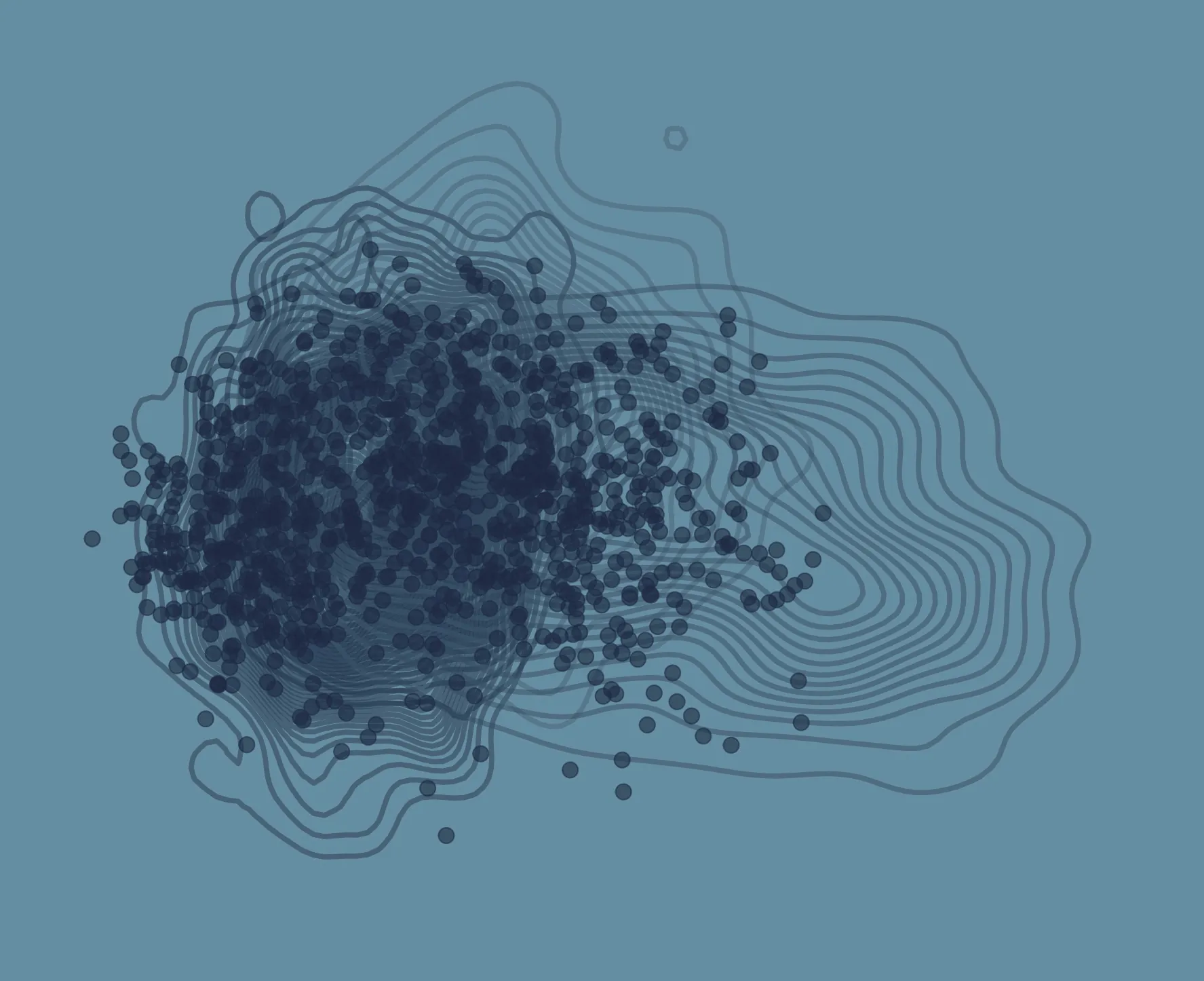
An abstracted figure from the Baliga Lab’s Science Advances publication depicting simulated states of core regulatory network topologies.
Cancer treatments are undergoing a revolution with the recent advent of targeted therapies, a class of medications that homes in on specific mutations or other molecular features of cancer cells. But these therapies don’t work for every patient, and many patients who see their cancer initially respond to targeted treatment will eventually relapse. Scientists at ISB developed Systems Genetics Network AnaLysis, or SYGNAL, to bring the promise of targeted cancer therapy to more patients in need. This computational, systems biology approach to cancer therapeutics identifies crucial genetic and other molecular changes in tumors with the goal of guiding real-time treatment decisions in the clinic.
- Funded by National Institutes of Health, National Science Foundation, National Cancer Institute Department of Energy, Washington Research Foundation, Celgene, Bristol Myers Squibb, various foundations
- Led by Nitin Baliga, MSc, PhD
- Key collaborators:
- Charles Cobbs, MD, Swedish Neuroscience Institute
- Parvinder Hothi, PhD, Swedish Neuroscience Institute
- Anoop Patel, MD, Duke University
SYGNAL: A Systems Biology Approach to Personalized Cancer Treatment
One of cancer’s hallmarks is its ability to rapidly mutate its DNA: Cancer cells contain hundreds, if not thousands, of DNA mutations that are not found in the patient’s healthy cells. This set of mutations is different from patient to patient and even from one cancer cell to another in the same patient. A very small percentage of these mutations are what are known as “driver mutations,” meaning they are essential for the cancer’s survival. In addition to mutations, cancer cells can escape treatments with epigenetic changes, modifications to a cell’s DNA that do not change the underlying genetic code. If researchers and clinicians could see consequences of a patient’s unique set of driver mutations and epigenetic mechanisms in a snapshot, they’d have a much better chance of matching an effective treatment to that patient — and course-correcting with new treatments when the cancer changes over time.
Delineating consequences of these epigenetic and genetic drivers is a classic systems biology problem, says SYGNAL’s lead researcher, Nitin Baliga, PhD. Baliga and his team at ISB are using SYGNAL, a computational method to identify consequences of a tumor’s genetic and epigenetic drivers, in studies of the brain cancer glioblastoma and the blood cancer multiple myeloma. By taking a holistic view of the genes and molecules involved in cancer growth and progression, the scientists are able to identify gene networks important for an individual patient’s cancer at any given time. It’s akin to Google Maps’ ability to give you personalized driving directions based on real-time traffic and road closure information, Baliga says.
Instead of traffic data, SYGNAL relies on information about a tumor’s genetic sequences and what is known as its transcriptome, the full readout of which genes are switched on in a given cell. The team is now using this approach to understand how glioblastoma and multiple myeloma develop treatment resistance and, ultimately, how to overcome that resistance to deliver more powerful and personalized treatments to patients.
Understanding cancer recurrence in glioblastoma
The most common form of brain cancer, glioblastoma is very aggressive and nearly always becomes resistant to standard treatments like chemotherapy and radiation. Baliga and colleagues are working together with collaborators from the Swedish Neuroscience Institute in Seattle to understand the mechanisms behind this acquired resistance, focusing on a rare and stubborn group of cells within tumors called glioma stem-like cells (GSCs). GSCs are notorious for their resistance to drugs like temozolomide, the standard chemotherapy treatment for glioblastoma. GSCs can transition into different cell states, which helps them evade therapy and acquire resistance after treatment. The team is using SYGNAL to study how glioma stem cells become resistant during the course of cancer treatment and whether existing cancer drugs, developed for other types of cancer, might work to kill these cells before they gain resistance and drive cancer recurrence. By using cells and tissue slices taken directly from glioblastoma patients who donate biopsies to research, the scientists hope to find principles of drug resistance and recurrence in glioblastoma that could inform future clinical trials of personalized medicine for this cancer.
The ISB group is also collaborating with scientists at Duke University, using patient-derived “xenografts,” avatars of an individual patient’s tumors grown in mice, as well as data taken directly from glioblastoma patients’ tumors. This approach allows the team to apply SYGNAL to gain a more complete picture of glioblastoma’s evolution by following cells in the same patient from initial diagnosis through treatment, as well as in the intervening timeframe using their tumor avatars in the animal model, to map the gene networks that drive treatment resistance and recurrence in an individual patient.
Uncovering the biology of multiple myeloma relapse
Unlike glioblastoma patients, multiple myeloma patients currently have many treatment options available to them, many of which can extend patients’ lives significantly. But even with so many newly developed therapies, this cancer remains incurable: Patients nearly always see recurrences of their cancer despite initially responding to therapies. ISB researchers are using SYGNAL to uncover the gene networks that drive disease progression and underlie the development of treatment resistance in multiple myeloma. By understanding the molecular machinery that drives resistance and relapse, the researchers hope to better enable oncologists in providing personalized care to multiple myeloma patients and even identify new therapy targets to prevent relapses.
Citations
- Park JH, Hothi P, de Lomana ALG, Pan M, Calder R, Turkarslan S, Wu WJ, Lee H, Patel AP, Cobbs C, Huang S, Baliga NS. Gene regulatory network topology governs resistance and treatment escape in glioma stem-like cells. Sci Adv. 2024. doi: 10.1126/sciadv.adj7706.
- Turkarslan S, He Y, Hothi P, Murie C, Nicolas A, Kannan K, Park JH, Pan M, Awawda A, Cole ZD, Shapiro MA, Stuhlmiller TJ, Lee H, Patel AP, Cobbs C, Baliga NS. An atlas of causal and mechanistic drivers of interpatient heterogeneity in glioma. medRxiv. 2024. doi: 10.1101/2024.04.05.24305380.
- Park JH, de Lomana ALG, Marzese DM, Juarez T, Feroze A, Hothi P, Cobbs C, Patel AP, Kesari S, Huang S, Baliga NS. A Systems Approach to Brain Tumor Treatment. Cancers (Basel). 2021. doi: 10.3390/cancers13133152.
- Park JH, Feroze AH, Emerson SN, Mihalas AB, Keene CD, Cimino PJ, de Lomana ALG, Kannan K, Wu WJ, Turkarslan S, Baliga NS, Patel AP. A single-cell based precision medicine approach using glioblastoma patient-specific models. NPJ Precis Oncol. 2022. doi: 10.1038/s41698-022-00294-4.
- Wall MA, Turkarslan S, Wu WJ, Danziger SA, Reiss DJ, Mason MJ, Dervan AP, Trotter MWB, Bassett D, Hershberg RM, Lomana ALG, Ratushny AV, Baliga NS. Genetic program activity delineates risk, relapse, and therapy responsiveness in multiple myeloma. NPJ Precis Oncol. 2021. doi: 10.1038/s41698-021-00185-0.
- Plaisier CL, O’Brien S, Bernard B, Reynolds S, Simon Z, Toledo CM, Ding Y, Reiss DJ, Paddison PJ, Baliga NS. Causal Mechanistic Regulatory Network for Glioblastoma Deciphered Using Systems Genetics Network Analysis. Cell Syst. 2016. doi: 10.1016/j.cels.2016.06.006.
- Toledo CM, Ding Y, Hoellerbauer P, Davis RJ, Basom R, Girard EJ, Lee E, Corrin P, Hart T, Bolouri H, Davison J, Zhang Q, Hardcastle J, Aronow BJ, Plaisier CL, Baliga NS, Moffat J, Lin Q, Li XN, Nam DH, Lee J, Pollard SM, Zhu J, Delrow JJ, Clurman BE, Olson JM, Paddison PJ. Genome-wide CRISPR-Cas9 Screens Reveal Loss of Redundancy between PKMYT1 and WEE1 in Glioblastoma Stem-like Cells. Cell Rep. 2015. doi: 10.1016/j.celrep.2015.11.021.
- Plaisier CL, Baliga NS. Harnessing the power of human tumor-derived cell lines for the rational design of cancer therapies. Pigment Cell Melanoma Res. 2012. doi: 10.1111/j.1755-148X.2012.01020.x.
- Plaisier CL, Bare JC, Baliga NS. miRvestigator: web application to identify miRNAs responsible for co-regulated gene expression patterns discovered through transcriptome profiling. Nucleic Acids Res. 2011. doi: 10.1093/nar/gkr374.
- Plaisier CL, Pan M, Baliga NS. A miRNA-regulatory network explains how dysregulated miRNAs perturb oncogenic processes across diverse cancers. Genome Res. 2012. doi: 10.1101/gr.133991.111.
Technologies Developed
SYGNAL (SYstems Genetics Network AnaLysis); MINER (Mechanistic Inference of Node-Edge Relationships)
Spinout Companies
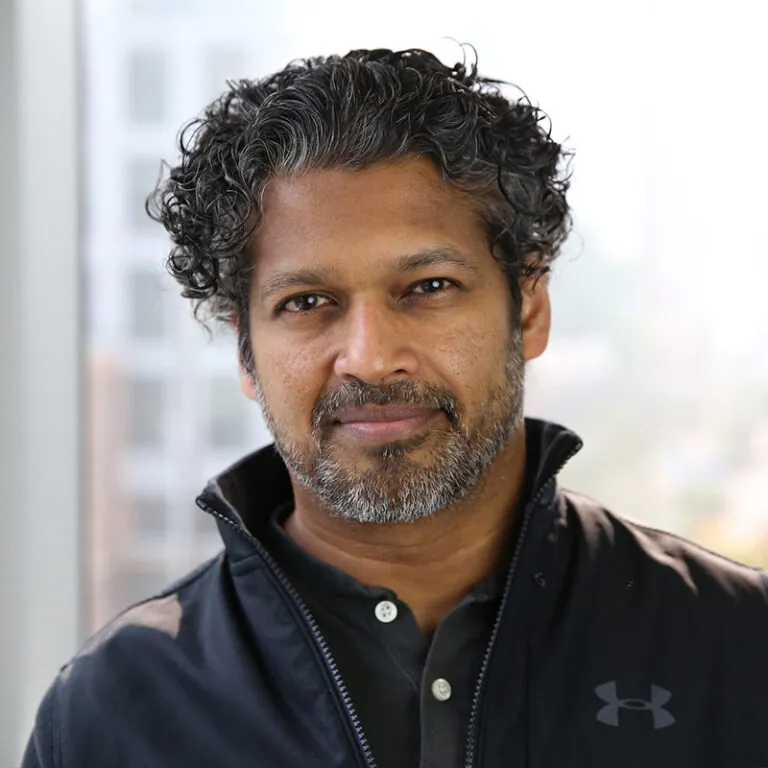
Contact Dr. Nitin Baliga
Senior Vice President, Director and Professor
ISB